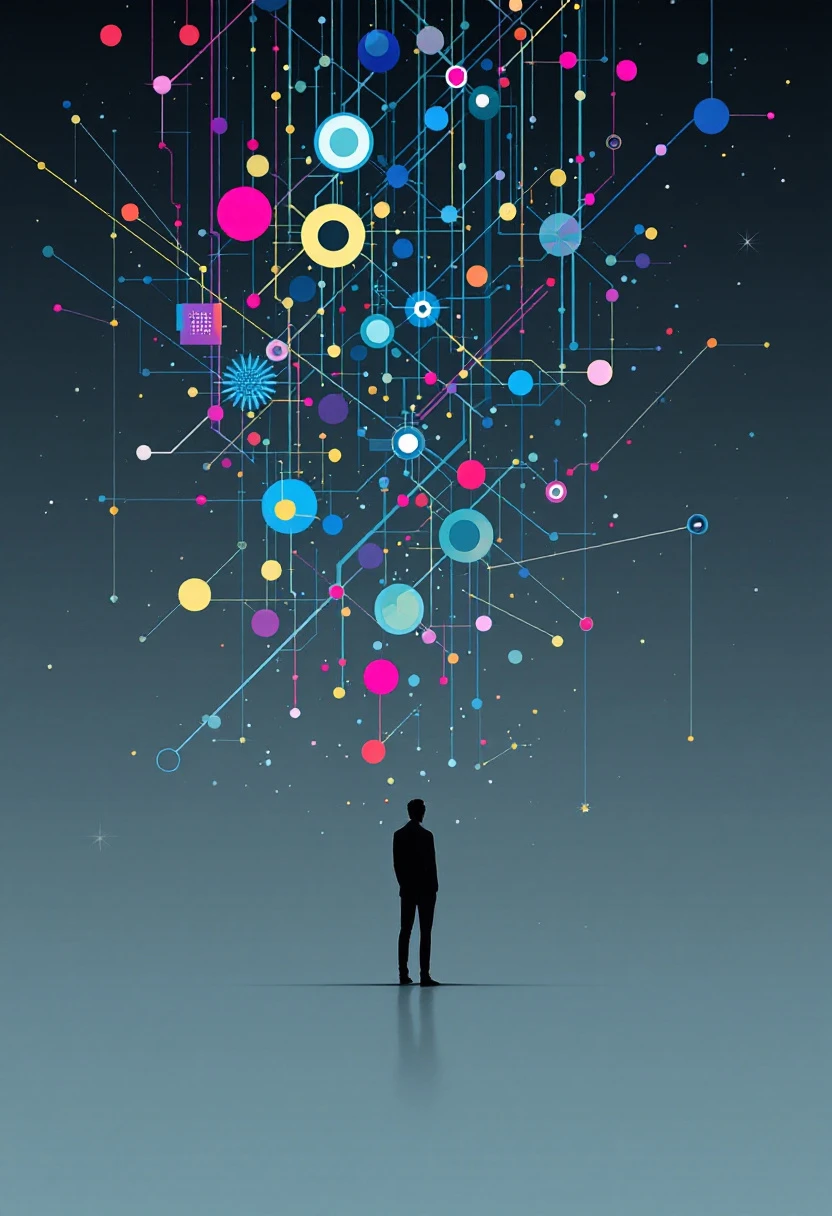
Concept Erasure
The removal or suppression of specific concepts or features from a model's internal representation, primarily to enhance its interpretability or rectify unwanted biases in its outputs.
Concept erasure in AI refers to the technique of eliminating specific concepts or features from a model's internal representations, typically neural networks, to increase interpretability, enhance privacy, or reduce biases that may lead to unethical decisions. This process involves identifying and surgically altering the internal states or pathways within a model that correspond to these concepts, without significantly affecting the model's overall performance. By doing so, researchers and practitioners aim to gain insights into a model's decision-making process, ensure that sensitive or protected attributes do not influence decisions disproportionately, and comply with regulatory standards regarding fairness and transparency. Additionally, concept erasure is pertinent in applications where the presence of certain features could lead to privacy invasions or discriminatory actions, thus aligning AI system outputs more closely with ethical considerations and legislative frameworks.
The term "concept erasure" began to gain traction around 2020, following advancements in understanding neural network interpretability and a growing emphasis on AI ethics and fairness. This period marked an increased focus on developing techniques to manipulate and analyze model internals to ensure compliant and transparent AI systems.
Key contributors to the development of concept erasure include researchers like Finale Doshi-Velez, Been Kim, and their colleagues, who have been pivotal in exploring model interpretability and the mechanisms by which AI systems can be made fairer and more comprehensible. Their work often intersects with interdisciplinary efforts involving computer science, ethics, and law, contributing significantly to the theoretical and practical advancements in this area.