Autonomous Sustainability Monitoring
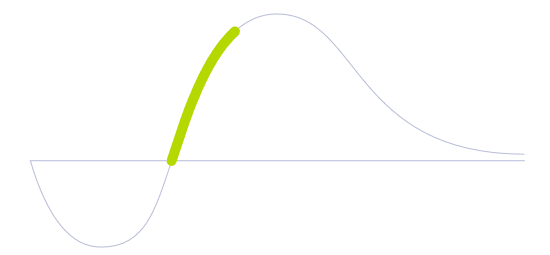
Technology Life Cycle
Marked by a rapid increase in technology adoption and market expansion. Innovations are refined, production costs decrease, and the technology gains widespread acceptance and use.
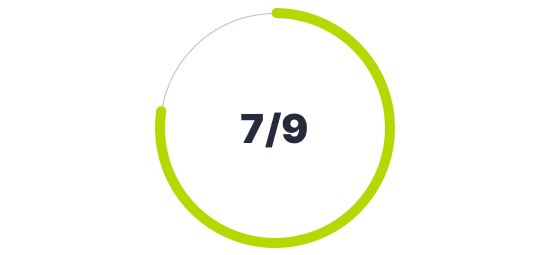
Technology Readiness Level (TRL)
Prototype is fully demonstrated in operational environment.
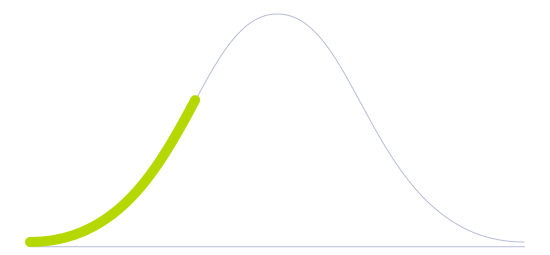
Technology Diffusion
Embrace new technologies soon after Innovators. They often have significant influence within their social circles and help validate the practicality of innovations.
Also known as Automated Environmental Monitoring, this technology uses sensors, satellite imagery, machine learning, and artificial intelligence (AI) to collect and analyze data related to sustainability in real-time. It helps businesses and government organizations to measure, track, and optimize their environmental impact, energy consumption, and resource usage. This technology is being applied in various sectors, from commercial buildings and industrial facilities to agricultural settings and transportation fleets.
This solution works by deploying a network of sensors and data collection devices throughout a facility, location, or equipment, which capture real-time data on energy use, water consumption, waste production, greenhouse gas emission, and other sustainability metrics. The data is then transmitted to a centralized platform where machine learning algorithms and AI models process it and generate insights and recommendations for optimizing sustainability performance. Emerging systems might also include satellite imagery that provides sustainability metrics.
The primary benefit of Autonomous Sustainability Monitoring is that it helps organizations to identify areas where they can reduce their environmental impact and save resources while also improving operational efficiency and reducing costs. For example, it can identify areas where energy is being wasted or where water usage is higher than necessary and provide recommendations for reducing consumption.
Additionally, the technology can provide live alerts and notifications when sustainability metrics exceed predetermined thresholds, allowing organizations to take immediate action to prevent or mitigate potential sustainability issues. It can also generate reports and dashboards that provide detailed insights into sustainability performance over time, which can be used to track progress and demonstrate accountability to stakeholders.
Future Perspectives
Instead of working as a single application solely reserved for companies, this technology could function as a collective monitoring platform for social and environmental impact by tackling global environmental challenges. For that, AI initiatives are creating open-source tools and APIs to provide models and the base infrastructure for enterprises that want to make their businesses greener and hold themselves more accountable.
Considering that all of these tools are available for free, governments and small initiatives could take advantage of this software as a means to pursue accountability and how their practices fit into global objectives in terms of sustainability and global warming. In combination with other technologies and systems such as blockchain and the internet of things, these initiatives could spread in smart cities and add a new layer of sustainable accountability to urban spaces too. Finally, this technology might be able to analyze entire industrial segments, making it easier to create a future of industrial ecology and foster more circular economic practices. This could help companies be viewed as more trustworthy in the eyes of consumers.
Image generated by Envisioning using Midjourney