Synthetic Data
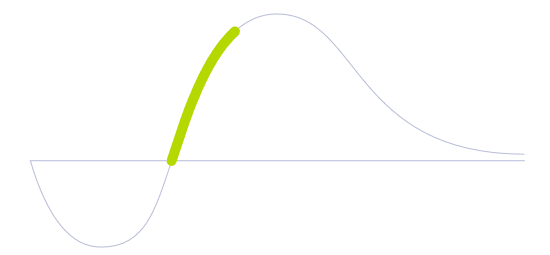
Technology Life Cycle
Marked by a rapid increase in technology adoption and market expansion. Innovations are refined, production costs decrease, and the technology gains widespread acceptance and use.
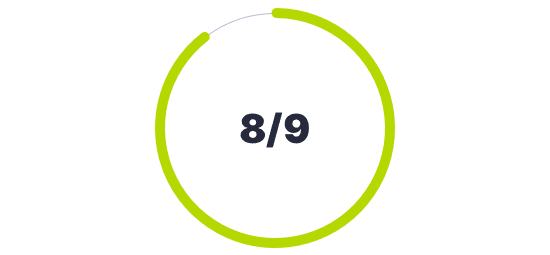
Technology Readiness Level (TRL)
Technology is developed and qualified. It is readily available for implementation but the market is not entirely familiar with the technology.
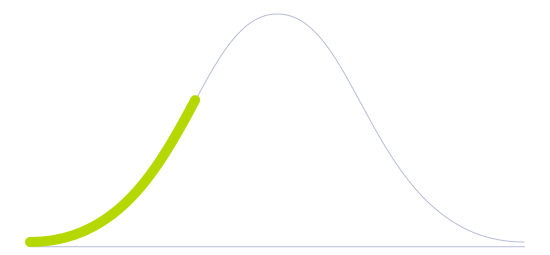
Technology Diffusion
Embrace new technologies soon after Innovators. They often have significant influence within their social circles and help validate the practicality of innovations.
Synthetic Data refers to artificially generated data sets, enabling privacy-friendly Big Data innovation. These artificial data sets are based on original data that often include personal details collected from sources like CRM databases, financial transactions, medical records, or smart city data.
Existing real-world data is used to train a synthetic data engine in a secure IT environment such as a private cloud, SaaS contexts, or within premises. In the engine, deep neural networks then automatically identify and understand patterns, structures, and correlations, even in vast and complex data sets. When training is complete, the software can generate unlimited synthetic data sets, retaining the statistical properties of the original data source. Some alternative techniques include semantic approaches, generative adversarial networks, and statistically rigorous sampling from real data.
Synthetic Data can be used for training AI models, product demos, hackathons, scenario simulations, internal prototyping, advanced analytics, development and testing, data monetization, and open innovation, as sharing data with third parties no longer poses privacy concerns. It is also compliant with GDPR and other data protection regulations, as customer identification becomes impossible. It also supports smaller companies, startups, and academia to innovate in a world where Big Data is concentrated in the hands of Big Tech. Applications can be seen across different sectors, such as finance, insurance, healthcare, government, mobility, and telecommunications.
This solution allows for more privacy-compliant, scalable, faster, and less expensive access to enhanced data, as opposed to real data, which is often expensive, biased, imbalanced, unavailable, or unusable due to privacy regulations. It also overcomes a flaw of classic data anonymization techniques, such as data destruction, where the reidentification of individual customers is still possible, even with the few remaining data points.
Future Perspectives
One of the main hurdles to applying Big Data strategies and AI training models lies in privacy concerns. Synthetic Data has the potential to democratize Big Data and AI systems while protecting individual privacy and flourishing innovation across sectors. In the future, Synthetic Data could overshadow real data in training models and could become the new norm. Access to artificial datasets could also allow academia and small and medium businesses to create powerful innovations and compete with Big Tech, creating more diversity of solutions and perspectives.
Image generated by Envisioning using Midjourney